Systems and sensors that use AI-supported software increase the service life and operational reliability of networked assets, but this is not possible without associated cybersecurity. Ideally, holistic systems are placed at three central levels. And this is already possible today.
Energy network operators and asset managers already have to compensate for the growing loss of personnel knowledge and the increasing maintenance requirements of aging operating equipment. At the same time, challenges and burdens are increasing:
The urgent expansion of grids, the feed-in of renewable energies and the decentralization of energy supply in the face of ever increasing energy demands hardly seem manageable. A key role is therefore being played by new technologies that help monitor and diagnose assets. Such intelligent data-driven systems make it possible to use the existing infrastructure more efficiently and extend its service life. The perfect interaction of all automation levels is crucial for this: from the sensor to the data node on the transformer to the level of global asset management.
“This is no longer a dream of the future; it is already possible today,” explains Tobias Gruber, MR product manager who is involved in the development of algorithms and mathematical training methods. “Reinhausen already offers a large portfolio of self-learning sensors and systems. And their capabilities are constantly increasing through mutual networking and an ever-growing learning curve.” The digitalization of assets is a necessary step for the reasons mentioned. But this transformation process — the independent further development of the systems themselves – will bring about changes in all industries, and also bring benefits that are not yet imaginable today. Since it is obvious that AI is here to stay, it makes sense to use it to support the energy industry at all levels.

“Reinhausen already offers a large portfolio of self-learning sensors and systems. And their capabilities are constantly increasing through mutual networking and an ever-growing learning curve.”
Tobias Gruber, Maschinenfabrik Reinhausen
At the process level, various sensors record numerous signals — reliably and precisely — directly at the transformer and forward them to a central communication node at the field level for consolidation. This provides reliable information on the maintenance and health status of the respective transformers. These evaluations then come together at the control level where maintenance strategies for the entire fleet can be developed. However, this only works if the sensor technology is modularly expandable and manufacturer-independent so that it can react agilely to future needs in fleet maintenance and renewal. And with such a high level of data exchange between the levels, cybersecurity is of particular importance.
There is more to the term cybersecurity than just protection against hacker attacks.
Security expert Dr. Hubert Feyrer explains exactly what this means in an interview.


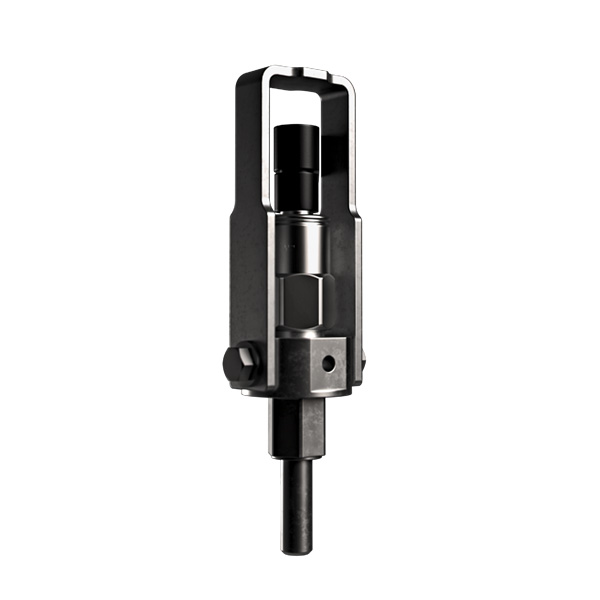
1. INTELLIGENT SENSOR TECHNOLOGY
Sensors collect information – that much is obvious. Smart sensors can also evaluate it. But what does that mean in concrete terms? Which data can be better evaluated using machine learning than with previous methods? An important standard measure, for example, is the analysis of the insulating oil in the gas phase (DGA – dissolved gas analysis). In all common analysis methods, the gas is extracted, which exposes it each time to fluctuating external factors such as ambient temperature, air pressure, humidity and much more. Ensuring that these values always remain constant for measurement would consume vast sums of money and significantly increase the complexity of DGA systems.
This is where artificial intelligence comes into play. Using training data sets, the algorithm in the smart DGA system learns the relationships between the gas concentration in the insulating oil, the sensor signal and the possible disturbing influences. With the aid of mathematical-statistical methods from the machine learning toolbox, the DGA sensor is thus “trained” during its development phase. The new intelligent specialist is then installed on the transformer and immediately knows what to do. “With the laboratory analysis of the oil as a reference point, the DGA sensor then also learns about its transformer and continuously recalibrates itself,” says Gruber. “In this way, effects such as sensor drift, aging of the insulating oil and the like can be compensated for, and consistent measurement repeatability ensured.”
Gas analysis for on-load tap-changers looks a bit more difficult, because in contrast to the transformer, fewer data sets on gas formation rates and typical gas concentrations have been developed. “Analyzing on-load tap-changers requires a lot of expert knowledge about how the particular tap-changer type works and how it operates,” Gruber tells us. That’s why it doesn’t just come down to analyzing the key gases. Rather, in this regard, statistical-mathematical algorithms use a much broader data set. In addition to gas concentrations, information about the on-load tap-changer such as the number of switching operations, the oil quantity, or the time since the last oil change are also used as input variables. The result is a diagnosis with an indication of probabilities, which is far ahead of pure gas analysis.
“Analyzing on-load tap-changers requires a lot of expert knowledge about how the particular tap-changer type works and how it operates.”
Tobias Gruber, Maschinenfabrik Reinhausen
For a more comprehensive assessment of on-load tap-changers, MSENSE® VAM® is the online diagnostic tool that performs a vibro-acoustic measurement by detecting vibrations that occur during the switching process of an on-load tap-changer. The associated algorithm represents the switching operation in a limit value curve, which is calculated more precisely with each switching operation. Thus, the system iteratively learns during the switching operations what the acoustic signature of a correctly operating on-load tap-changer looks like. “And it works everywhere,” Gruber explains, “for new as well as for existing on-load tap-changers of all manufacturers and designs.”
One thing that no one wants to find in their transformer is moisture. In addition to many possible test points, it is above all the breakdown voltage of the insulating oil that gives concrete indications of possible contamination. However, the methods proposed by current standards for analyzing this voltage are cumbersome and expensive. Here, too, online monitoring by self-learning algorithms is better: They learn the relationship between relative oil moisture, oil temperature and breakdown voltage. In this process, data for breakdown voltage levels at different oil temperatures and humidities are determined experimentally using a reference method, e.g. IEC 60156, and these data are then used to train a mathematical model. The model is validated and optimized using test data that is independent of the training data and again, a trained artificial expert is on hand to reliably identify where problems might be brewing.



2. INTELLIGENT DATA EVALUATION
Monitoring systems on power transformers already play a major role, especially in terms of stable fault detection. However, this alone is not enough. They continuously report information and data which must be evaluated and interpreted in relation to each other – and this for every transformer. This is no easy task, especially when several sensors are capable of the same function. “For example, if temperature, partial discharge and DGA sensors are all capable of detecting winding faults, their statements are generally not comparable which can result in contradictory statements, and a simple diagnosis is not possible.”
That’s why at MR all sensors are integrated into the ETOS® and evaluated by the self-learning algorithms of the ISM® computing unit within the ETOS®. “A Bayesian network checks which fault patterns best match the warnings that occur – and don’t occur. In addition, a priori probabilities for typical transformer faults and sensor reliability are considered.” The result is a probability estimate for all known transformer problems, with the most likely problems displayed to the customer along with a list of reasons for the findings. This allows the professional to interpret the diagnostic results in a simplified and efficient manner. “The Bayesian network Asset Intelligence for power transformers provides a guide to minimize risk and take corrective action quickly,” says Tobias Gruber.

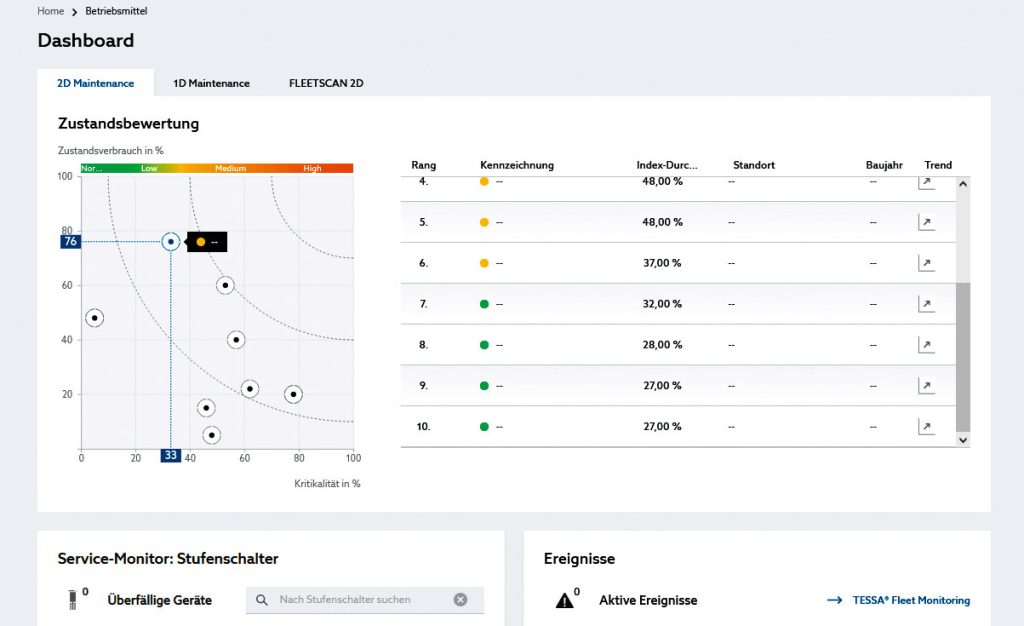
3. INTELLIGENT FLEET MANAGEMENT
Most transformer fleets currently consist of multiple products that come from various suppliers and differ in age and equipment. Asset management must do justice to them all. The best way to do this is with a central solution that uses and evaluates all online and offline data – from sensor data to manually entered measured values following an on-site inspection. Reinhausen has developed TESSA® APM® for this purpose. The software behind the platform evaluates data, recognizes trends and independently issues recommendations for action. In the process, the intelligent software continuously learns during operation and “gets to know” its connected assets. Because at some point the relevant specialist may be missing and it will be TESSA® APM® that says, for example, “Take a look at transformer 5A, it’s a bit sensitive with the oil”.
The fact that the software combines all data, can be used remotely and warns prophylactically in the event of malfunctions, saves asset managers valuable time and also fills the personnel supply gap. A triad of intelligent sensors at the process level, data evaluation at the field level on the transformer, and fleet management at the control level thus prepare network operators and OEMs for the challenges of today and tomorrow.
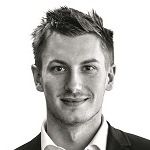
YOUR CONTACT
Do you have questions about MR’s AI solutions?
Tobias Gruber is there for you:
T.Gruber@reinhausen.com